AI-Powered SERS Enables Rapid and Accurate Detection of Virus Co-Infections
- Yiping Zhao
- Jan 29
- 2 min read
A new study from the University of Georgia presents a groundbreaking diagnostic tool combining Surface-Enhanced Raman Spectroscopy (SERS) and deep learning to rapidly detect respiratory virus co-infections. Published in ACS Sensors, this method offers a fast, scalable, and cost-effective alternative to traditional virus detection techniques.
The Challenge of Virus Co-Infections
Co-infections, where multiple viruses infect a patient simultaneously, can complicate treatment and increase disease severity. During the COVID-19 pandemic, studies showed that co-infections increased mortality from 6% to 16%, highlighting the urgent need for better detection. Current methods like PCR and sequencing are precise but costly and time-consuming, limiting their use in fast-paced medical settings.
Our research team developed a diagnostic system that uses SERS to detect virus-specific molecular signals and a deep learning model, MultiplexCR, to classify and quantify them. By analyzing over 1.2 million SERS spectra from 11 single viruses and multiple mixed-virus samples, the model achieved a classification accuracy of 98.6% and a mean absolute error of 0.028 in concentration prediction, outperforming conventional methods. This method offers the following features:
Speed: Results in just 15 minutes, far faster than PCR.
Accuracy: 98%+ classification precision, enhancing diagnostic reliability.
Cost-Effectiveness: At $10–$30 per test, it's significantly cheaper than PCR and sequencing.
Scalability: Easily expandable to detect emerging viral strains.
Point-of-Care Suitability: Compact and efficient for real-time, on-site diagnostics.
Beyond virus detection, this AI-SERS system has potential applications in disease surveillance, environmental monitoring, and food safety. Its adaptability makes it a promising tool for future diagnostic advancements.
Further validation using real clinical samples and comparisons with PCR will be key to its adoption in mainstream healthcare. The adaptability of MultiplexCR suggests its usefulness in detecting other biomolecular signatures, broadening its impact across multiple fields.
For more details, check out the full study in ACS Sensors.
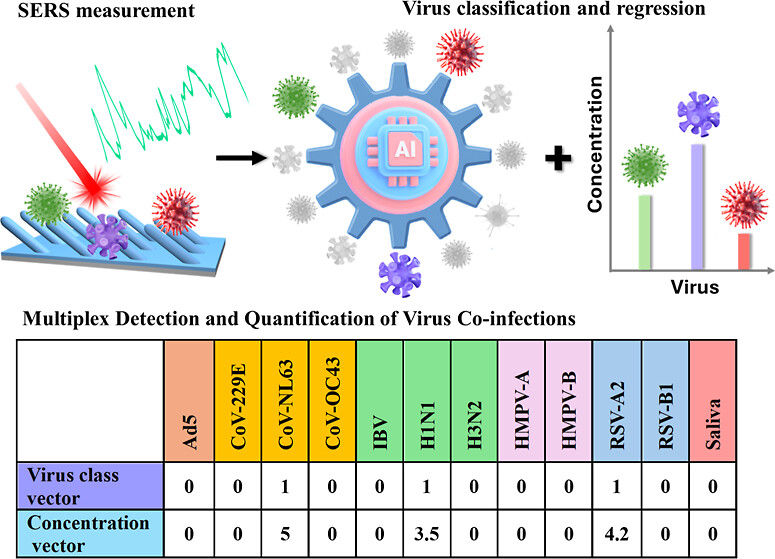
Comments