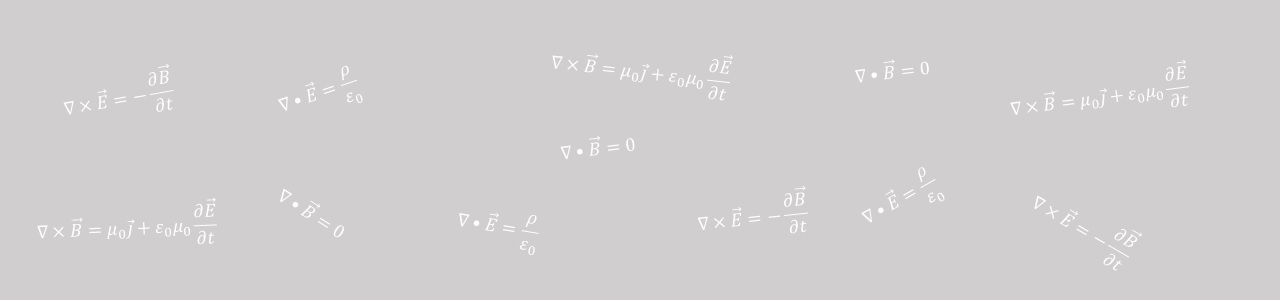
Machine Learning
Machine learning (ML) is revolutionizing sensor development and material design by leveraging data-driven approaches to achieve unprecedented advancements in predictive accuracy, operational efficiency, and scalability. ML models enable the extraction of subtle patterns from complex datasets, enhancing the performance of sensors and materials for a wide range of applications. In ours group, ML is seamlessly integrated with experimental and theoretical frameworks, addressing key challenges in sensing, material innovation, and environmental monitoring. By combining artificial intelligence with advanced nanostructures and spectroscopic techniques, the group is pushing the boundaries of what is possible in these fields.
In collaboration with Profs. Xianyan Chen, Wenzhan Song, Ping Ma, and Bin Ai, the group focuses on developing diverse machine learning and deep learning models to classify and quantify viruses in various media, detect bacterial mycotoxins and biomarkers, and analyze Per- and Polyfluoroalkyl Substances (PFAS) and other spectral data. In partnership with Prof. Bin Ai's group, the team employs an inverse design strategy to create plasmonic nanostructures capable of producing specific colors. They also integrate phase space reconstruction with convolutional neural networks to significantly enhance the predictive accuracy and performance of plasmonic hydrogen sensors. In addition, we are creating an open-source spectroscopy platform called SpectraGuru, designed to empower the community with tools for accessible, efficient, and innovative spectral analysis. These interdisciplinary efforts showcase the transformative potential of ML in advancing sensor technologies and material science for critical applications in health, energy, and environmental sustainability.
​
Publication List
-
Joshua C. Rothstein, Jiaheng Cui, Yanjun Yang, Xianyan Chen, and Yiping Zhao, “Ultra-Sensitive Detection of PFASs using Surface Enhanced Raman Scattering and Machine Learning: A Promising Approach for Environmental Analysis,” Sensors & Diagnostics 3, 1272 - 1284 (2024).
-
Amit Kumar, Md Redwan Islam, Susu M. Zughaier, Xianyan Chen and Yiping Zhao, “Precision Classification and Quantitative Analysis of Multiple Bacteria Biomarkers via Surface-Enhanced Raman Spectroscopy and Machine Learning,” Spectrochimica Acta A 320, 124627 (2024).
-
Yanjun Yang, Jiaheng Cui, Dan Luo, Jackelyn Murray, Xianyan Chen, Sebastian Hülck, Ralph Tripp, and Yiping Zhao, “Rapid Detection of SARS-CoV-2 Variants Using ACE2-Based SERS Sensor Enhanced by CoVari Deep Learning Algorithms,” ACS Sensors 9, 3158 – 3169 (2024).
-
Xiangxin Lin, Mingyu Cheng, Xinyi Chen, Jinglan Zhang, Yiping Zhao, and Bin Ai, “Unlocking Predictive Capability and Enhancing Sensing Performances of Plasmonic Hydrogen Sensors via Phase Space Reconstruction and Convolutional Neural Networks,” ACS Sensors 9, 3877 - 3888 (2024).
-
Yanjun Yang, Hao Li, Les Jones, Jackelyn Murray, Hemant Naikare, Yung-Yi C. Mosley, Teddy Spikes, Sebastian Hülck, Ralph A. Tripp, Bin Ai, and Yiping Zhao, “Advancing SERS Diagnostics in COVID-19 with Rapid, Accurate, and Label-Free Viral Load Monitoring in Clinical Specimens via SFNet Enhancement,” Advanced Materials Interfaces, DOI 10.1002/admi.202400013.
-
Yangxiu Chen, Yanjun Yang, Jiaheng Cui, Hong Zhang, and Yiping Zhao, “Decoding PFAS contamination via Raman spectroscopy: A combined DFT and machine learning investigation,” J. Harz. Mater. 465, 133260 (2024).
-
Yilong Ju, ​Oara Neumann, ​Mary Bajomo, ​Yiping Zhao, ​Peter Nordlander, ​Naomi J. Halas, and ​Ankit Patel, “Identifying Surface-Enhanced Raman Spectra with a Raman Library Using Machine Learning,” ACS Nano 17, 21251–21261 (2023).
-
​Chun Liu, Jinglan Zhang, Yiping Zhao, and Bin Ai, “Inverse Design of Plasmonic Nanohole Arrays by Combing Spectra and Structural Color in Deep Learning,” Advanced Intelligent Systems, 2300121 (2023).
-
Yanjun Yang, Hao Li, Les Jones, Jackelyn Murray, James Haverstick, Hemant K. Naikare, Yung-Yi C. Mosley, Ralph A Tripp, Bin Ai, Yiping Zhao, “Rapid detection of SARS-CoV-2 RNA in human nasopharyngeal specimens using surface-enhanced Raman spectroscopy and deep learning algorithms,” ACS Sensors 8, 297 - 307 (2023).
-
Mary M. Bajomo, Yilong Ju, Jingyi Zhou, Simina Elefterescu, Corbin Farr, Yiping Zhao, Oara Neumann, Peter Nordlander, Ankit Patel, and Naomi J. Halas, “Computational chromatography: a machine learning strategy for demixing individual chemical components in complex mixtures,” Proc. Natl. Acad. Sci. U.S.A. 119, e2211406119 (2022).
-
Yanjun Yang, Beibei Xu, Jackelyn Murray, James Haverstick, Xianyan Chen, Ralph A. Tripp, and Yiping Zhao, “Rapid and quantitative detection of respiratory viruses using surface-enhanced Raman spectroscopy and machine learning,” Biosensors and Bioelectronics 217, 114721 (2022).
-
Yanjun Yang, Beibei Xu, James Haverstick, Nabil Ibtehaz, Artur MuszyÅ„ski, Xianyan Chen, Muhammad E. H. Chowdhury, Susu M. Zughaier, and Yiping Zhao, “Differentiation and classification of bacterial endotoxins based on surface enhanced Raman scattering and advanced machine learning,” Nanoscale 14, 8806-8817 (2022).